Exploring the Role of Artificial Intelligence in Cyber Security
- Andrew Harper
- Aug 11, 2023
- 6 min read
Updated: Jun 6, 2024
Every 39 seconds, there’s a cyber-attack somewhere in the world. In the age of rapid technological advancements, this alarming statistic underscores a sobering reality - our digital life is more vulnerable than ever.
With artificial intelligence (AI) increasingly becoming the backbone of many technological processes, its intersection with cybersecurity has never been more pronounced. Imagine it as not just a tool but a sophisticated ally (or adversary) that continually progresses, learns, and adapts.
In this piece, we will dwell on understanding the impact of this technology on cybersecurity approaches.
What is the connection between cybersecurity and AI?
IDC projected global spending on AI technology to surpass $500 billion in 2023, highlighting the critical importance of AI integration. As this technology advances, cybersecurity has become a central focus in discussions. The growing nature of cybersecurity engagement is driven by aggressive threats that leverage AI and machine learning to target enterprises.
Use of AI in cyber security
Detection and analysis of threats. AI-powered cybersecurity systems excel in detecting and analysing possible threats. AI systems can reveal strange patterns or behaviours suggestive of assaults by continually tracking network operations and analysing massive volumes of data. This allows for faster and more precise danger detection, which reduces response times and potential harm.
Automated response to incidents. Automation capabilities of AI improve incident response in cybersecurity. When danger is recognized, artificial intelligence in security can launch automatic operations to limit or mitigate the assault, reducing the need for manual involvement and reaction time. This rapid response is critical for avoiding widespread harm or hacking of data.
Analytics for predictive cybersecurity. Predictive cybersecurity analytics are made possible by AI’s data analysis skills. AI can foresee likely attacks and weaknesses by analysing massive datasets and spotting trends, allowing companies to take preventative actions to bolster their security posture.
Advantages and disadvantages of AI in cybersecurity
Efficient risk management. AI revolutionises how organisations tackle security threats. Combining vast amounts of data, it pinpoints vulnerabilities that might escape human notice.
Streamlined regulatory compliance. Fulfilling regulatory mandates, be it GDPR, HIPAA, or PCI-DSS, becomes more manageable with AI. Systems fortified with artificial intelligence can autonomously monitor for compliance, detect potential breaches, and craft reports in line with stipulated standards.
Increased false alarms. A notable disadvantage of AI in cybersecurity is its susceptibility to false positives. Since AI-driven security models grow from historical data, novel threats that don’t align with known patterns might trigger unnecessary alarms. This over-alertness can swamp security personnel, leading to oversight of actual threats.
Talent shortfall. Incorporating AI in cybersecurity calls for a unique blend of expertise. Professionals need to be adept at crafting, deploying, and overseeing these AI systems. Unfortunately, the industry currently grapples with a dearth of such seasoned experts.
Elevated costs. The transition to AI-centric security models might be financially draining for organisations, especially those with constrained budgets. These systems demand specialised hardware, sophisticated software solutions, and a team of experts for optimal operation.
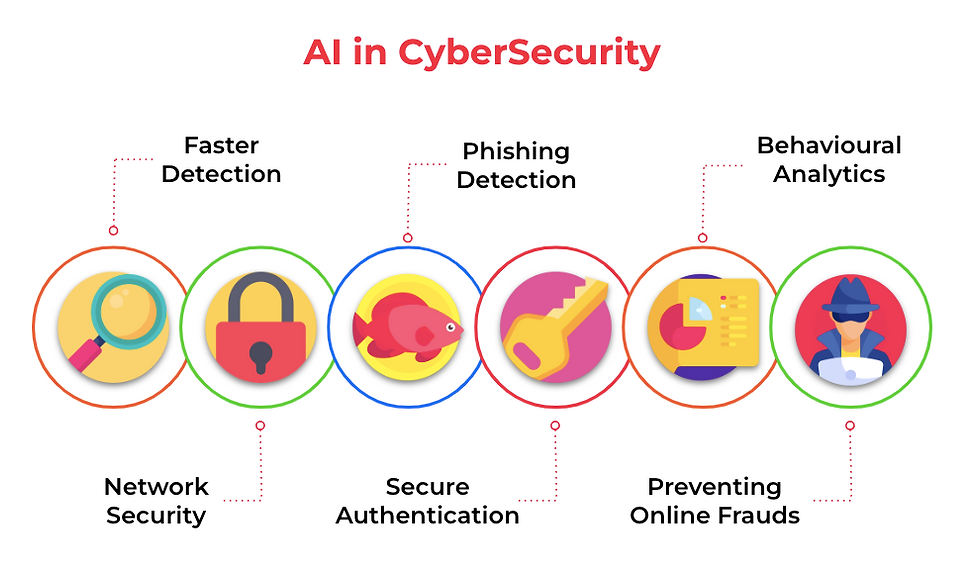
AI-Powered threat detection and prevention
Every day, about 500,000 new malware cases are discovered. One of the most critical functions of AI cybersecurity solutions is detecting and preventing attacks. It enables businesses to battle cyber invasions by analysing threat patterns and detecting unexpected activity. AI systems may filter through massive volumes of data, such as network traffic, logs, and other security-related occurrences, to identify potential risks. Furthermore, it has the unique capacity to detect and neutralise unexpected threats that traditional security measures may miss.
Applications of AI in Cybersecurity
Using AI for cybersecurity helps identify irregularity in datasets by training machine learning models on standard patterns. Once done, these models flag anomalies in new data. This method is pivotal for uncovering discreet threats that penetrate networks.
Even with the most covert tactics, differences from typical behaviour emerge, which anomaly detection models can capture. By aggregating network traffic and employing AI and ML, these systems establish norms for user, device, and application behaviours, known as User and Entity Behavior Analytics (UEBA). Real-time traffic is then assessed against these baselines to pinpoint and alert about any deviations.
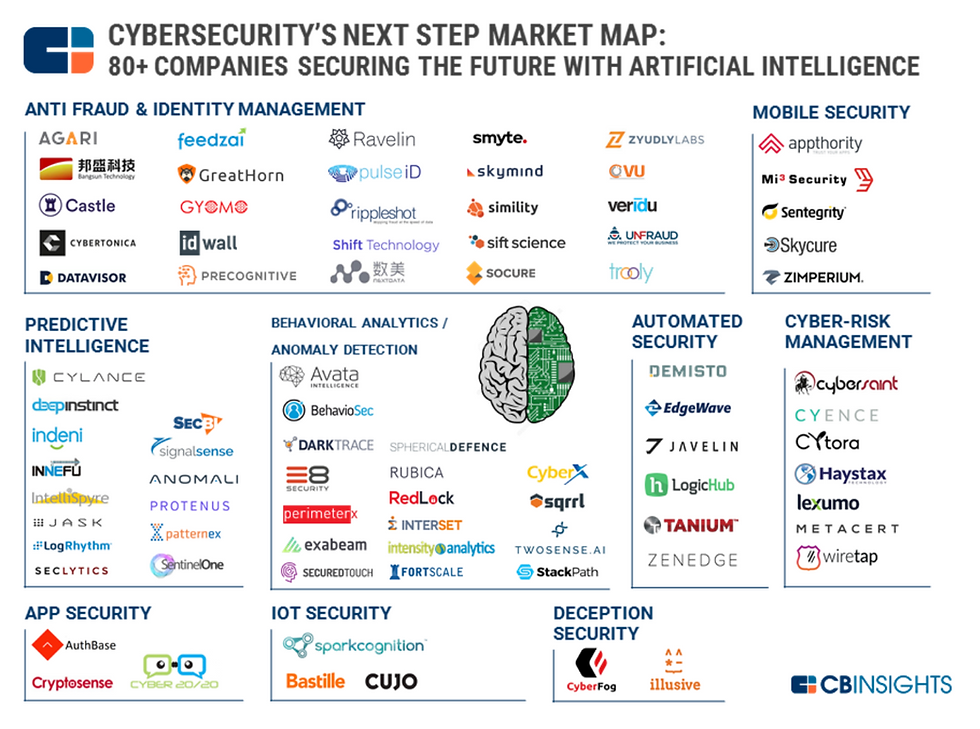
Source: CBINSIGHTS
Behavioural analysis
AI is used to assess user activity within a company’s network. AI can detect variations in user behaviour that may signal insider threats or compromised accounts by comprehending usual user actions. This analysis aids in the strengthening of access controls and the prevention of unwanted entry.
Machine Learning
A subset of AI, machine learning, enables security systems to collect information from past data and adjust to new threats promptly. AI algorithms may recognize typical network activity patterns; any divergence from these patterns can be highlighted as a danger. This feature is valuable for detecting zero-day attacks and other previously undiscovered risks.
Real-world examples of AI in cybersecurity
The UK’s Energy Saving Trust is committed to cutting the nation’s carbon emissions by 80% by 2050. Recognizing the complexity of their network, they prioritised enhancing cybersecurity to safeguard client data and intellectual assets. They partnered with Darktrace, a leading British-American IT firm focused on cybersecurity, to introduce real-time threat detection. Unlike traditional systems that rely on set rules or signatures, Darktrace’s platform is self-learning and identifies unusual behaviours autonomously. Consequently, the trust’s security team gains instant network insights and is promptly notified of potential cyber threats.
Sogeti Luxembourg’s security experts, part of a renowned global IT consultancy, faced challenges in swiftly analysing the massive volume of threat data they encountered. In response, Sogeti integrated IBM’s QRadar, an AI-powered security intelligence tool capable of evaluating 10,000 events every second. It empowers their cybersecurity team to tackle threats more rapidly and assuredly. Thus, threat information that took three hours is now accessible in just three minutes.
Advancements in AI for cybersecurity
The most recent advances in artificial intelligence have been beyond all expectations. A Gartner poll predicts a $62 billion investment in AI software in 2022, indicating the tremendously unexplored potential of this type of technology.
Autonomous AI systems & self-learning algorithms in cybersecurity
These systems offer a multitude of benefits to the realm of cybersecurity. Their ability to function at a speed that far surpasses human capabilities allows for the immediate address of threats through real-time defence mechanisms.
The data surge in this current era poses challenges, but AI is well-equipped to manage this. It processes vast amounts of information from countless sources simultaneously, preventing the data deluge from becoming unmanageable. Additionally, the inherent adaptability of self-learning algorithms stands out. They adjust and fine-tune their defences as threats develop, ensuring continuous protection against new vulnerabilities.
There’s a tangible danger in becoming overly reliant on AI. Organisations might inadvertently downplay the significance of human intuition and expertise, potentially neglecting vital components of a comprehensive cybersecurity strategy. Moreover, the quest for accuracy can sometimes lead AI systems astray, causing them to identify harmless activities as threats mistakenly. Perhaps the most challenging is the opaque decision-making in specific AI models. For instance, the complexity of deep learning algorithms can be hard to decipher, leading to concerns about transparency and the ramifications of decisions made in a virtual “black box.”
AI and its vulnerabilities to adversarial attacks
The very existence of adversarial attacks unveils concerning vulnerabilities within AI frameworks. When contextualised within the domain of cybersecurity, the stakes rise significantly. Armed with the knowledge of these vulnerabilities, malicious entities might exploit them, avoiding the defences set up by AI-driven security protocols.
Fortifying security against adversarial onslaughts:
Continuous model retraining. The digital sphere is in perpetual flux, and AI models must mirror this dynamism. Companies can enhance their ability to identify and defend against adversarial interferences by regularly updating and retraining them.
Defensive distillation. This method involves training the AI model to generate more generalised or “smoothed” outputs. The rationale? Eliminating the sharp distinctions in output interpretations makes it more challenging for attackers to pinpoint and exploit vulnerabilities.
Input scrutiny. Before processing any data, examining inputs for any anomalies or irregularities is prudent. Such precautionary checks can help spot and thwart adversarial efforts early in their tracks.
Summary
The fusion of AI and cybersecurity is reshaping digital defence strategies. While AI offers accelerated threat detection and response, it also presents challenges like false alarms and potential misuse by adversaries. Balancing the power of AI with ethical and transparent practices is paramount. In a world where cyberattacks are almost routine, the approach to AI-driven cybersecurity should be informed, proactive, and always ethically grounded. Stay alert and invested in a secure future of AI in cyber security.